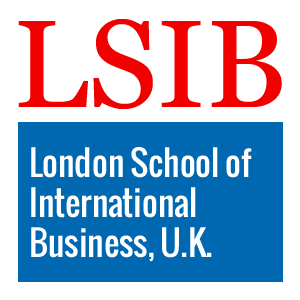
Home / AI-Driven Data Analysis for Engineers: Avoid Common Mistakes
As you delve into the world of AI-driven data analysis as an engineer, it's crucial to be aware of common mistakes that can hinder your success. Let's uncover these pitfalls and learn how to avoid them to ensure your projects are a resounding success.
One of the most common mistakes engineers make in AI-driven data analysis is overlooking the quality of the data they are working with. Without high-quality data, your analysis will be flawed and produce inaccurate results.
Mistake | Solution |
---|---|
Not cleaning or preprocessing data adequately | Ensure data is clean, complete, and free of errors before analysis |
Ignoring outliers or missing values | Address outliers and missing data appropriately |
Selecting the right model is crucial for accurate data analysis. Choosing the wrong model can lead to inaccurate predictions and conclusions.
Mistake | Solution |
---|---|
Using a complex model when a simpler one would suffice | Select the simplest model that fits the data adequately |
Ignoring the assumptions of the chosen model | Ensure the model assumptions are met before analysis |
By avoiding these common mistakes and following best practices in AI-driven data analysis, you can elevate your projects to new heights of success. Stay vigilant, prioritize data quality, and make informed decisions to ensure your analysis is accurate and insightful.