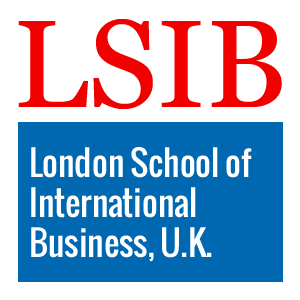
Home / AI-Based Malware Sandbox Detection: Avoid Common Mistakes
As a participant in the Professional Certificate in AI-Based Malware Sandbox Detection, it is crucial to be aware of common mistakes that can undermine the effectiveness of malware sandbox detection. By understanding these mistakes and learning how to avoid them, you can enhance your skills and improve your ability to detect and mitigate AI-based malware attacks.
Mistake | Description |
---|---|
1. Insufficient Feature Selection | Failing to choose relevant features for AI-based detection can lead to inaccurate results and missed malware threats. |
2. Overfitting the Model | Overfitting occurs when a model is trained too closely on specific data, leading to poor generalization and reduced effectiveness in detecting new malware variants. |
3. Ignoring Explainability | Failing to prioritize explainability in AI models can hinder understanding of detection mechanisms and make it challenging to interpret results. |
Here are some strategies to help you avoid common mistakes in AI-based malware sandbox detection:
By being aware of these common mistakes and implementing strategies to avoid them, you can strengthen your expertise in AI-based malware sandbox detection and enhance your ability to defend against sophisticated cyber threats effectively.