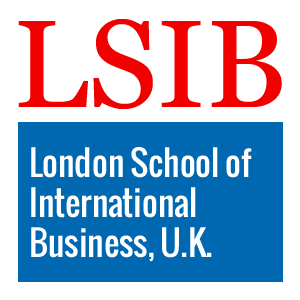
Home / Avoiding Mistakes in AI-Based Audit Evaluation: Effective Strategies
One of the most common mistakes in AI-based audit evaluation is the lack of proper data management. It is essential to ensure that the data used in the evaluation process is accurate, reliable, and up to date. Without proper data management, the results of the audit evaluation may be compromised.
To avoid this mistake, organizations should implement a data governance framework that sets guidelines for data collection, storage, and usage. This framework will help ensure that the data used in AI-based audit evaluation is of high quality and meets the necessary standards.
Another common mistake is the overreliance on AI algorithms to perform the audit evaluation without considering human judgment and expertise. While AI algorithms can streamline the process, they should not replace the critical thinking and analysis skills of auditors.
To avoid this mistake, organizations should combine AI technology with human expertise. Auditors should work alongside AI algorithms to ensure a more comprehensive and accurate audit evaluation.
Ignoring ethical considerations in AI-based audit evaluation can lead to biased or discriminatory results. It is crucial to consider the ethical implications of using AI technology in the audit process and ensure that the evaluation is fair and unbiased.
Organizations should incorporate ethical guidelines into their AI-based audit evaluation process. This includes ensuring transparency, accountability, and fairness in the evaluation process to mitigate any ethical concerns.
Failing to regularly update AI models used in audit evaluation can lead to outdated results and inaccurate insights. It is essential to continually refine and improve AI models to ensure they remain effective and relevant.
To avoid this mistake, organizations should implement a process of continuous monitoring and optimization of AI models. This includes regularly updating data sets, refining algorithms, and incorporating new technologies to enhance the audit evaluation process.
Common Mistakes | Effective Strategies |
---|---|
Lack of Proper Data Management | Implement a Data Governance Framework |
Overreliance on AI Algorithms | Combine AI with Human Expertise |
Ignoring Ethical Considerations | Incorporate Ethical Guidelines |
Failure to Regularly Update AI Models | Continuous Monitoring and Optimization |